Recent advancements in Remote Sensing (RS) technologies, driven by higher spectral, spatial, and temporal resolution, have resulted in larger and more diverse data volumes. This has created significant challenges in efficiently processing and analyzing data for Earth Observation (EO) applications. Concurrently, the rise of Deep Learning (DL) techniques, particularly computer vision models with complex architectures, has highlighted the need for parallel algorithms to ensure scalability. As a result, high-performance computing (HPC) has become essential in addressing the increasing demands of data-intensive RS and geoscience tasks. The rapid evolution of AI technologies, including transformers and self-supervised learning, is revolutionizing how researchers extract meaningful insights from remote sensing data. These models excel in tasks such as object detection, segmentation, and pattern recognition, addressing key challenges like limited labeled data. HPC infrastructure is crucial to realize the full potential of these technologies in operational EO applications.
Speaker
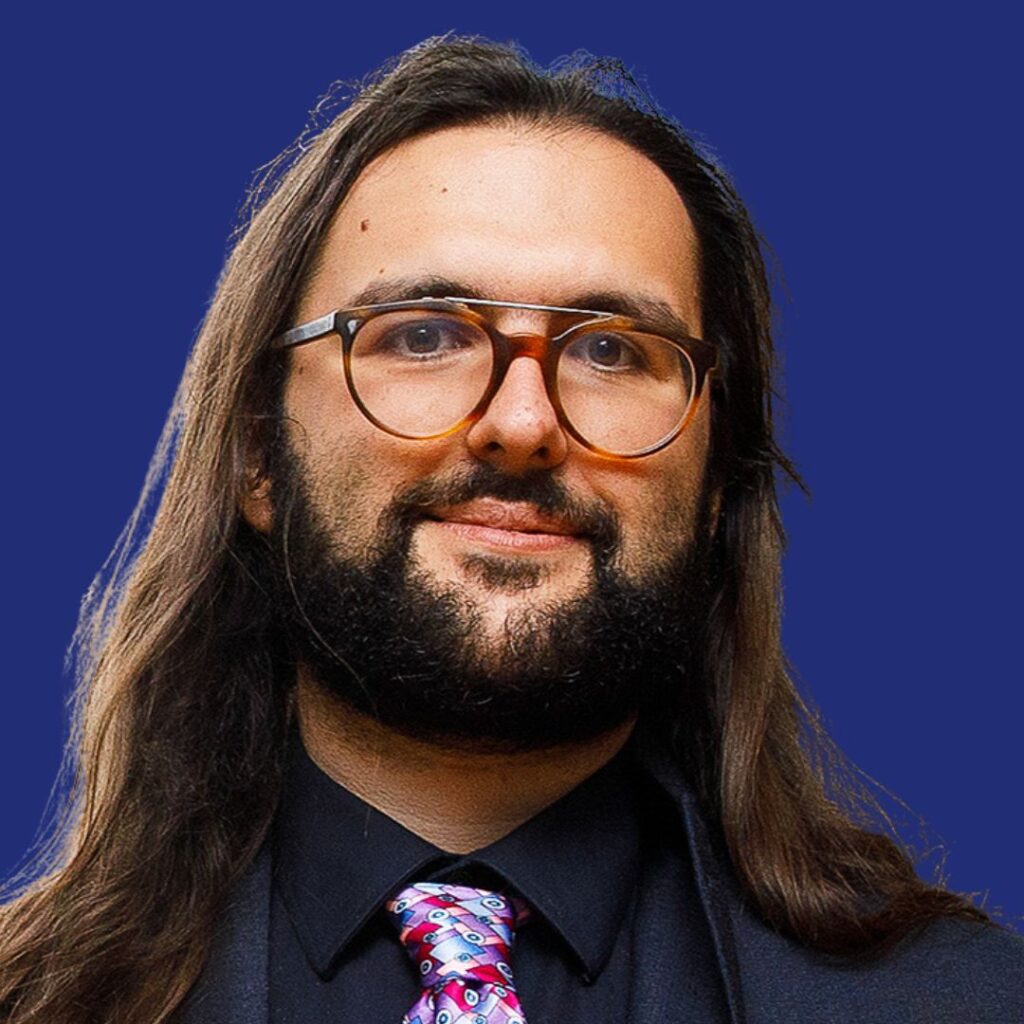
Rocco Sedona – Jülich Supercomputing Centre, Forschungszentrum Jülich
Rocco Sedona (Member, IEEE) received the B.Sc. and M.Sc. degrees in information engineering from the University of Trento, Trento, Italy, in 2016 and 2019, respectively, and the Ph.D. degree in computational engineering from the University of Iceland, Reykjavik, Iceland, in 2023.
He is a deputy head of the “AI and ML for Remote Sensing” Simulation and Data Lab, JSC, Germany. His research interests primarily lie in the field of deep learning and its application to remote sensing data at scale. He has extensively utilized optical satellite data acquired by Landsat (NASA) and Sentinel (ESA) missions toward near real-time land-cover classification. In addition, he specializes in distributed deep learning on high-performance computing systems, an area of study that he has been actively engaged in since 2019.
Event Timeslots (1)
Fri 20 – Applications & Performance Evaluation
-
R. Sedona (JSC Germany)